Towards Energy-Efficient Spiking Neural Networks: A Robust Hybrid CMOS-Memristive Accelerator
Document Type
Article
Publication Date
1-19-2024
Department
Department of Electrical and Computer Engineering
Abstract
Spiking Neural Networks (SNNs) are energy-efficient artificial neural network models that can carry out data-intensive applications. Energy consumption, latency, and memory bottleneck are some of the major issues that arise in machine learning applications due to their data-demanding nature. Memristor-enabled Computing-In-Memory (CIM) architectures have been able to tackle the memory wall issue, eliminating the energy and time-consuming movement of data. In this work we develop a scalable CIM-based SNN architecture with our fabricated two-layer memristor crossbar array. In addition to having an enhanced heat dissipation capability, our memristor exhibits substantial enhancement of 10% to 66% in design area, power and latency compared to state-of-the-art memristors. This design incorporates an inter-spike interval (ISI) encoding scheme due to its high information density to convert the incoming input signals into spikes. Furthermore, we include a time-to-first-spike (TTFS) based output processing stage for its energy-efficiency to carry out the final classification. With the combination of ISI, CIM and TTFS, this network has a competitive inference speed of 2μs/image and can successfully classify handwritten digits with 2.9mW of power and 2.51pJ energy per spike. The proposed architecture with the ISI encoding scheme can achieve ∼10% higher accuracy than those of other encoding schemes in the MNIST dataset.
Publication Title
ACM Journal on Emerging Technologies in Computing Systems
Recommended Citation
Nowshin, F.,
Hongyu, A.,
&
Yang, Y.
(2024).
Towards Energy-Efficient Spiking Neural Networks: A Robust Hybrid CMOS-Memristive Accelerator.
ACM Journal on Emerging Technologies in Computing Systems,
20(1).
http://doi.org/10.1145/3635165
Retrieved from: https://digitalcommons.mtu.edu/michigantech-p2/533
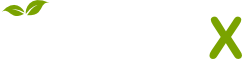
- Citations
- Citation Indexes: 2
- Usage
- Abstract Views: 20
- Captures
- Readers: 9
- Mentions
- News Mentions: 1