Document Type
Article
Publication Date
1-30-2023
Department
Department of Applied Computing; Department of Computer Science
Abstract
The rise of vision-based environmental, marine, and oceanic exploration research highlights the need for supporting underwater image enhancement techniques to help mitigate water effects on images such as blurriness, low color contrast, and poor quality. This paper presents an evaluation of common underwater image enhancement techniques using a new underwater image dataset. The collected dataset is comprised of 100 images of aquatic plants taken at a shallow depth of up to three meters from three different locations in the Great Lake Superior, USA, via a Remotely Operated Vehicle (ROV) equipped with a high-definition RGB camera. In particular, we use our dataset to benchmark nine state-of-the-art image enhancement models at three different depths using a set of common non-reference image quality evaluation metrics. Then we provide a comparative analysis of the performance of the selected models at different depths and highlight the most prevalent ones. The obtained results show that the selected image enhancement models are capable of producing considerably better-quality images with some models performing better than others at certain depths.
Publication Title
IEEE Access
Recommended Citation
Saleem, A.,
Paheding, S.,
Rawashdeh, N.,
Awad, A.,
&
Kaur, N.
(2023).
A Non-Reference Evaluation of Underwater Image Enhancement Methods Using a New Underwater Image Dataset.
IEEE Access.
http://doi.org/10.1109/ACCESS.2023.3240648
Retrieved from: https://digitalcommons.mtu.edu/michigantech-p/16866
Creative Commons License
This work is licensed under a Creative Commons Attribution 4.0 International License.
Version
Publisher's PDF
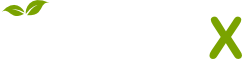
- Citations
- Citation Indexes: 17
- Usage
- Downloads: 672
- Abstract Views: 14
- Captures
- Readers: 29
- Mentions
- News Mentions: 1