Online predictive connected and automated eco-driving on signalized arterials considering traffic control devices and road geometry constraints under uncertain traffic conditions
Document Type
Article
Publication Date
3-2021
Department
Department of Civil, Environmental, and Geospatial Engineering
Abstract
For energy-efficient Connected and Automated Vehicle (CAV) Eco-driving control on signalized arterials under uncertain traffic conditions, this paper explicitly considers traffic control devices (e.g., road markings, traffic signs, and traffic signals) and road geometry (e.g., road shapes, road boundaries, and road grades) constraints in a data-driven optimization-based Model Predictive Control (MPC) modeling framework. This modeling framework uses real-time vehicle driving and traffic signal data via Vehicle-to-Infrastructure (V2I) and Vehicle-to-Vehicle (V2V) communications. In the MPC-based control model, this paper mathematically formulates location-based traffic control devices and road geometry constraints using the geographic information from High-Definition (HD) maps. The location-based traffic control devices and road geometry constraints have the potential to improve the safety, energy, efficiency, driving comfort, and robustness of connected and automated driving on real roads by considering interrupted flow facility locations and road geometry in the formulation. We predict a set of uncertain driving states for the preceding vehicles through an online learning-based driving dynamics prediction model. We then solve a constrained finite-horizon optimal control problem with the predicted driving states to obtain a set of Eco-driving references for the controlled vehicle. To obtain the optimal acceleration or deceleration commands for the controlled vehicle with the set of Eco-driving references, we formulate a Distributionally Robust Stochastic Optimization (DRSO) model (i.e., a special case of data-driven optimization models under moment bounds) with Distributionally Robust Chance Constraints (DRCC) with location-based traffic control devices and road geometry constraints. We design experiments to demonstrate the proposed model under different traffic conditions using real-world connected vehicle trajectory data and Signal Phasing and Timing (SPaT) data on a coordinated arterial with six actuated intersections on Fuller Road in Ann Arbor, Michigan from the Safety Pilot Model Deployment (SPMD) project.
Publication Title
Transportation Research Part B: Methodological
Recommended Citation
Zhao, S.,
&
Zhang, K.
(2021).
Online predictive connected and automated eco-driving on signalized arterials considering traffic control devices and road geometry constraints under uncertain traffic conditions.
Transportation Research Part B: Methodological,
145, 80-117.
http://doi.org/10.1016/j.trb.2020.12.009
Retrieved from: https://digitalcommons.mtu.edu/michigantech-p/14591
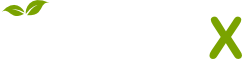
- Citations
- Citation Indexes: 25
- Usage
- Abstract Views: 34
- Captures
- Readers: 60
- Mentions
- News Mentions: 5
Publisher's Statement
© 2021 Elsevier Ltd. Publisher’s version of record: https://doi.org/10.1016/j.trb.2020.12.009