Forecasting time-to-failure of machine using hybrid Neuro-genetic algorithm – a case study in mining machinery
Document Type
Article
Publication Date
12-1-2016
Department
Department of Geological and Mining Engineering and Sciences
Abstract
Forecasting of time-to-failure is an important aspect of a mining machine for the performance assessment, fault detection and schedule maintenance. The knowledge of failure time allows more defined arrangement of preventive maintenance. Traditional methods, including lifetime distribution models, fault tree analysis and Markov models, have a limitation of assuming a specific statistical distribution function to fit the failure time data. In this study, a hybrid data-driven method using neural network and genetic algorithm is proposed to forecast failure time. The forecasting model was developed using neural network algorithm and all the neural network parameters, i.e. input nodes, hidden nodes and the learning algorithms, are selected automatically using the genetic algorithm. The developed model was validated using the failure data of a mining machine. A case study was conducted investigating a load-haul-dump machine (LHD) in the mining industry. Failure historical data for the LHD machine were collected, and cumulative failure time was calculated for time-to-failure forecasting. Study results demonstrate that the developed model performs satisfactory in the prediction of next failure time. A comparative study reveals that the proposed method performs better than existing methods.
Publication Title
International Journal of Mining, Reclamation and Environment
Recommended Citation
Paithankar, A.,
&
Chatterjee, S.
(2016).
Forecasting time-to-failure of machine using hybrid Neuro-genetic algorithm – a case study in mining machinery.
International Journal of Mining, Reclamation and Environment,
32(3), 182-195.
http://doi.org/10.1080/17480930.2016.1262499
Retrieved from: https://digitalcommons.mtu.edu/michigantech-p/1192
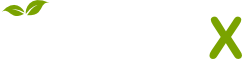
- Citations
- Citation Indexes: 14
- Usage
- Abstract Views: 4
- Captures
- Readers: 24
- Mentions
- News Mentions: 1